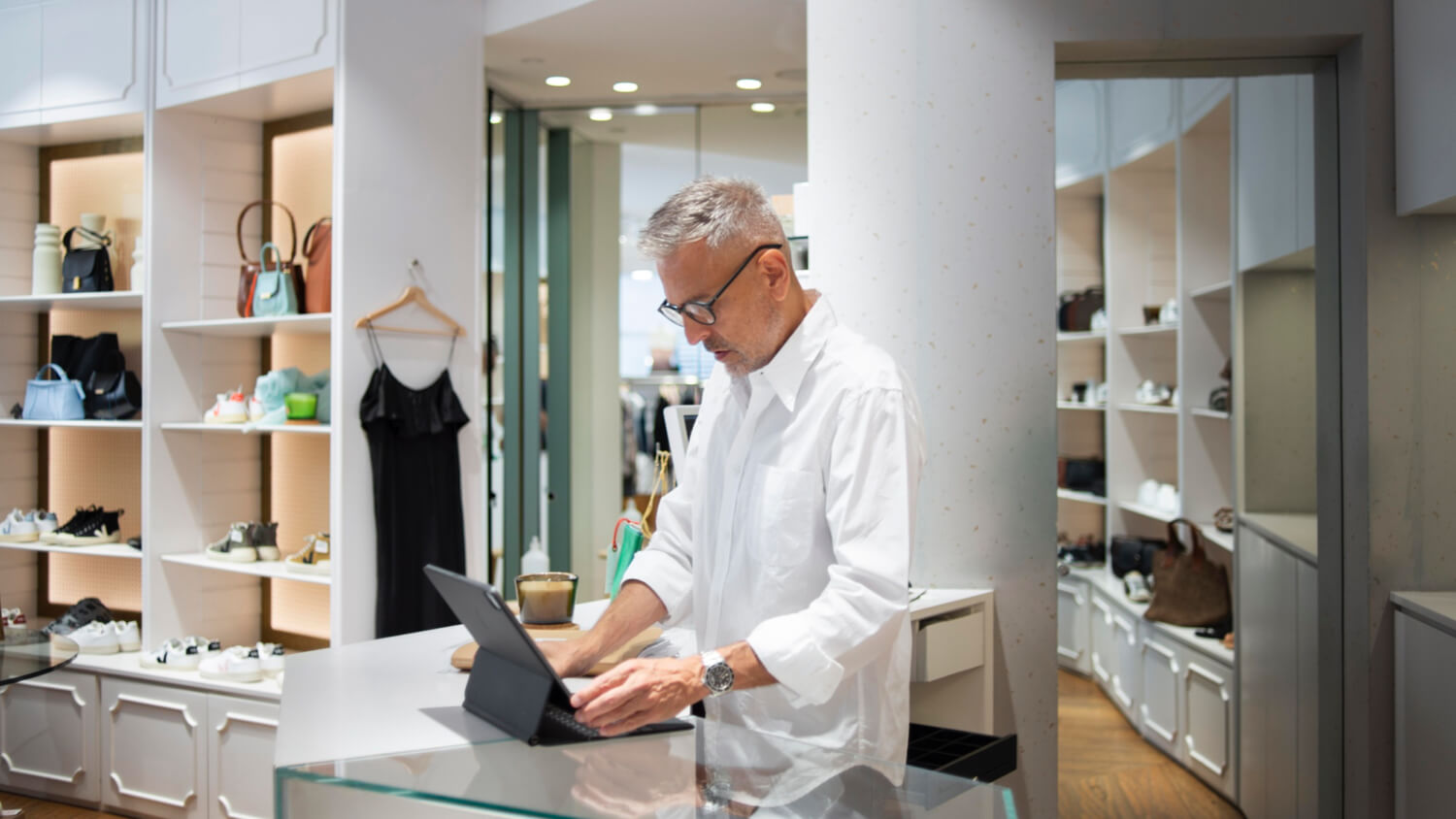
Unleashing the Power of Science and Data in Retail
Hatem Sellami is a serial entrepreneur who has successfully founded and co-founded multiple technology companies in the retail science space. Currently he is serving as the CEO of both RetSci and the software company, Cognira. We recently got together to talk about what exactly IS retail science.
A Brief History
So Hatem, obviously I know you’ve been doing this for 20 years but if you don’t mind kind of doing a little bit of an introduction. So what is your background with retail science and how did you get started with it?
I started in a company called Retek and at the time I was hired as a scientist on a science team that was responsible for building the forecasting engine to drive retail demand forecasting for large retailers. As a data scientist (back then we used to call them scientists) the role consisted of writing the code, developing the algorithms, and doing everything that would produce the engine that would drive the forecasts. Now, the demand forecast is basically trying to predict how many units will be sold at every store of every SKU that the retailer has. It is a “demand forecast” not a “sales forecast” because you want to know how many people will be coming in looking for that item. So a sales forecast is the demand forecast constrained by the availability of inventory. That’s the difference.The demand is unconstrained demand versus sales is constrained demand.
Back then in the late 90s, when we talked about retail science that was primarily demand forecasting. Demand forecasting is the most basic retail science component because everything in the retail operation depends on a good quality demand forecast. If you know the demand forecast then you know how many you have to order. You also know how many you need to put in the warehouse to be able to serve that demand. You also know how many people you need to have in the stores to work with the customers and serve that demand. There are so many things in the business operation that depend on the demand forecast and therefore it is very important that the demand forecast is as accurate as possible. As a result, science at that time, over 20 years ago, was primarily demand forecasting.
Eventually, we started dominating the space of demand forecasting. So then we started getting into other related areas that help retailers make better decisions leveraging science and data. That is what we call retail science today.
Retail Science Today
Basically today, every time you hear the word optimization in any process, that is typically driven by science. A great example is “markdown optimization.” For markdown optimization a retailer has inventory, usually the seasonal items they need to get rid of at the end of the season. They have leftover inventory so they want to get rid of it as quickly as possible. What they do is think “how can I maximize my revenue from this inventory and get rid of it by a specific date to give space to the new incoming products for the next season?”
So, I guess if your demand forecast wasn’t quite as accurate as you hoped you would end up with a situation where now you need markdown optimization?
The truth is that you’ll never get 100% accurate forecasts. Depending on what type of products you sell you’re going to have different accuracies for the forecast. Even the same retailer will have items where they get to 90% accuracy and they’ll have items where they will get 30% accuracy. If it is an item that you sell once a month or once every two months there is no way you can predict what day or what week even that item is going to be sold. Those very low volume items usually give you low quality forecasts. Meanwhile, high volume items that you sell a lot of every week are likely to give you higher accuracies.
Science in Fashion
Fashion, in particular, is very seasonal and the volumes for each unique item are very small. When you get to item and location and then size and then color you end up with very very low volumes for each SKU. At the end of the season, you start marking them down and that’s when you can use demand forecasting and science. Science helps you know whether you offer 10% off for four weeks or if you should go down to 20% if you have a lot more to get rid of. That is the optimization that needs to happen and there is a lot of science there to determine how to do that. Without data driven science support then retailers, merchants, or the buyer for that class or category will have to just pick a number and guess.
It is very hard to do manually because you must make decisions for every store. You end up deciding on one approach for the whole country. In some areas though, the item could be still selling. Why would you mark it down when you can still sell it at full price? That amount of detail is perfect for science to handle, but an individual does not have enough hours in the day to be able to handle it.
Forecasting as an Art
How often have you run into challenges where companies look at it more like an art?
That was especially true 20 years ago. Then the quality of the forecast was not great because there was not enough data. Also the systems and the algorithms were not as sophisticated. Systems were too expensive to have lots of computing power. The algorithms were simpler because we did not have enough computing power and data. There was skepticism back then. Over time, I think the systems have gotten better and we have more data. As a result, the forecasts are more accurate and there is more acceptance of the science.
Again, take the world of fashion where every season you’re bringing in new items that you have never sold in the past. It becomes very hard to predict those items and that’s really where the art comes into play. Planners will say “I know this summer red is going to be the hot color.” A system will never know that “red is going to be the hot color.” Another example is when you are opening a new store in a new city that you have never sold in before. How do I know how many items I will be selling? Or how many people will be coming to that store? Again, there are techniques that can be used to help mitigate those challenges and produce more accurate forecasts, but the goal is not to have a hundred percent accurate forecast because that is not achievable. The goal is to improve the forecast to the level where it really helps a retailer.
Just like when we worked with merchants early on, we still want to make sure that merchants understand that they have the power and the ability to change the forecasts. They should use the systems as a recommendation. The system will give you a lot of alerts and help you manage by exception. When you are not getting alerts about an item then that means that probably the forecast is going to be good. If I’m selling 100 units of an item every week in this particular store then the forecast is going to be 98, it’s going to be 110, it’s going to be 90. That is okay. Keep it. Let it go on auto pilot if you like. But the items where you have erratic sales, that you don’t know, that you have been selling 100 units and you get a forecast of 20, that is when something is wrong. Then the system will alert you as a user to those and you can go and check it out and make the adjustments.
We still want science to help and support the users and the merchants and the art. Not to replace the art. We want to figure out how to bring more data and decision support to make the art even better.
Other Areas For Retail Science
What other areas have you seen science applied really well?
Everything that merchants make decisions on in retail. Not just merchants either, supply chain and everyone. Certainly the area of markdown optimization. The area of pricing. Regular price optimization. Promotion and promotion optimization. That is what to promote, when to promote, how to promote, and all of those things.
Then, there is assortment optimization. How many styles do you want to bring in? How many colors do you want to bring in for a particular style? If you bring more colors in, then obviously each one of them will sell less. However, if you bring in less colors then you are bringing less options to your to your customers and they may not get the color they want. This is a very difficult problem that we have been making progress on over the years. We achieve that with assortment optimization.
Another area is workforce optimization. How do I optimize my workforce so that I don’t have people sitting in the store idle because there is nobody there? We will optimize where to assign people, to what stores, during which periods of the year and so on. Of course there is also supply chain and warehouse management. That is how to optimize where to position your warehouses. Where is each item stored for delivery based on demand? How to order, how many to order and when to order them and all that. That helps retailers avoid carrying too much inventory unnecessarily. Even in marketing and marketing mix there is a lot of science that is happening now in the industry. Modeling and optimizing marketing dollars and where they are spent.
Retail Science Throughout the Supply Chain
With better inventory management, has that actually created a challenge on the supplier side too? Have they now gotten to the point where they almost have to predict what the retailers are going to do who are in turn predicting what the consumers are going to do? Or has science not gotten down to the wholesale level?
No, the science has absolutely gotten to the wholesale level. In fact, for the wholesalers or distributors, or CPG vendors and suppliers the problems are a lot easier to handle because usually the volumes are bigger and the number of items is smaller. A typical retailer may have a thousand stores and they may have 50,000 SKUs. That is a lot of SKU store combinations. That means that they have to calculate a lot of forecasts every week.
Meanwhile, most suppliers usually have a very limited number of SKUs. They could have hundreds, they may have a few thousand. Also, they have fewer customers. When they sell to Target, they just need to know how many distribution centers Target has. That number may be 50 or 100. It is not going to be in the thousands. That is why the number of location combinations on the supply side is significantly smaller and the volumes are so much higher. It is because they are based on shipments where they group lots of items together so it becomes an easier problem to solve.
As a result, their forecasts are more accurate and they have been more receptive to forecasting and even price optimization or markdown and promotion optimization. I would say that they have been using science even longer than retailers.
Retail Science Internationally
What about internationally? Do you see the same applications of retail science internationally that you do in North America?
Definitely. Obviously there are certain parts of the world where retail is more advanced than others. For example, I worked with a retailer in South Africa where retail is very advanced. They are very innovative and I can tell you they are one of the most sophisticated retailers when it comes to forecasting algorithms and how to use forecasting to drive replenishment. And to drive other business operations. Western Europe and Australia, Eastern Europe even, are really the regions in the world where they have been adopting science in general.
Of course, in other parts of the world where organized retail is still developing it is probably going to take them a little while. Quite honestly though, many of those retailers are smaller retailers that don’t require advanced systems with highly scalable capabilities. In South America also, there are quite a few larger retailers that have adopted science.
Overall though, I think there’s still a long way to go. There are a lot of large, very successful retailers even here in the US that are not leveraging science in all the areas where they should be leveraging science.
Segments and Retail Science